Research progress of ultrasound, MRI imaging and imageomics in breast cancer
-
摘要: 乳腺癌是全球女性最常见的恶性肿瘤之一,发病率和致死率居癌症首位,早期诊断和早期治疗可以提高疾病预后效果,降低疾病相关死亡率。超声和MRI影像学是乳腺癌诊疗指南推荐对原发肿瘤评估无创检查方式,随着科技的发展,越来越多的影像学新技术应用于临床实践,乳腺癌检出率和诊断准确率都有了很大提高。人工智能的出现,拓展了影像组学的应用,推动了乳腺癌精准医疗的发展。本文针对超声和MRI影像学及影像组学在乳腺癌临床诊疗中的应用进展进行了综述。Abstract: Breast cancer is one of the most common malignant tumors in women in the world, with the highest incidence and mortality of cancer. Early diagnosis and treatment can improve the prognosis of the disease and reduce the disease-related mortality. Ultrasound and MRI imaging are the non-invasive examination methods recommended by the guidelines for the diagnosis and treatment of breast cancer for the evaluation of primary tumors. With the development of science and technology, more and more new imaging technologies are applied in clinical practice, and the detection rate and diagnostic accuracy of breast cancer have been greatly improved. The emergence of artificial intelligence has expanded the application of imaging omics and promoted the development of precision medicine for breast cancer. This article reviews the application of ultrasound and MRI imaging and imaging omics in clinical diagnosis and treatment of breast cancer.
-
Key words:
- breast cancer /
- ultrasound /
- magnetic resonance imaging /
- radiomics
-
表 1 乳腺癌分子分型
Table 1. Molecular typing of breast cancer
分型 指标 HER-2 ER PR Ki-67 HER-2阳性(HR阴性) + - - 任何 HER-2阳性(HR阳性) + + 任何 任何 三阴型(TN) - - - 任何 Luminal A型 - + +且尚表达 低表达 Luminal B型(HER-2阴性) - + 低表达或- 高表达 -
[1] Sung H, Ferlay J, Siegel RL, et al. Global cancer statistics 2020: GLOBOCAN estimates of incidence and mortality worldwide for 36 cancers in 185 countries[J]. CA Cancer J Clin, 2021, 71(3): 209- 49. doi: 10.3322/caac.21660 [2] 赫捷, 陈万青, 李霓, 等. 中国女性乳腺癌筛查与早诊早治指南(2021, 北京)[J]. 中国肿瘤, 2021, 30(3): 161-91. [3] Sala E, Mema E, Himoto Y, et al. Unravelling tumour heterogeneity using next-generation imaging: radiomics, radiogenomics, and habitat imaging[J]. Clin Radiol, 2017, 72(1): 3-10. doi: 10.1016/j.crad.2016.09.013 [4] 中国临床肿瘤学会. 乳腺癌诊疗指南(2022)[M]. 北京: 人民卫生出版社, 2022. [5] Sigrist RMS, Liau J, Kaffas AE, et al. Ultrasound elastography: review of techniques and clinical applications[J]. Theranostics, 2017, 7(5): 1303-29. doi: 10.7150/thno.18650 [6] Giuseppetti GM, Martegani A, Di Cioccio B, et al. Elastosonography in the diagnosis of the nodular breast lesions: preliminary report[J]. Radiol Med, 2005, 110(1/2): 69-76. [7] Shiina T, Nightingale KR, Palmeri ML, et al. WFUMB guidelines and recommendations for clinical use of ultrasound elastography: part 1: basic principles and terminology[J]. Ultrasound Med Biol, 2015, 41(5): 1126-47. doi: 10.1016/j.ultrasmedbio.2015.03.009 [8] Ricci P, Cantisani V, Ballesio L, et al. Benign and malignant breast lesions: efficacy of real time contrast-enhanced ultrasound vs. magnetic resonance imaging[J]. Ultraschall Med, 2007, 28(1): 57-62. doi: 10.1055/s-2006-927226 [9] 刘娟, 马苏美, 王惠. 自动乳腺超声成像系统的临床应用现状[J]. 中国医学影像学杂志, 2019, 27(8): 634-6, 640. doi: 10.3969/j.issn.1005-5185.2019.08.017 [10] Schiaffino S, Gristina L, Tosto S, et al. The value of coronal view as a stand-alone assessment in women undergoing automated breast ultrasound[J]. Radiol med, 2021, 126(2): 206-13. doi: 10.1007/s11547-020-01250-7 [11] Brunetti N, de Giorgis S, Zawaideh J, et al. Comparison between execution and reading time of 3D ABUS versus HHUS[J]. La Radiol Med, 2020, 125(12): 1243-8. doi: 10.1007/s11547-020-01209-8 [12] 韩晓蓉, 梁琦, 宁平, 等. 超声光散射成像乳腺诊断系统对T1期乳腺癌的诊断价值[J]. 中华乳腺病杂志: 电子版, 2019, 13(6): 343-9. doi: 10.3877/cma.j.issn.1674-0807.2019.06.005 [13] Bae MS, Seo M, Kim KG, et al. Quantitative MRI morphology of invasive breast cancer: correlation with immunohistochemical biomarkers and subtypes[J]. Acta Radiol, 2015, 56(3): 269-75. doi: 10.1177/0284185114524197 [14] Liu HB, Zhan HW, Sun D, et al. Comparison of BSGI, MRI, mammography, and ultrasound for the diagnosis of breast lesions and their correlations with specific molecular subtypes in Chinese women[J]. BMC Med Imaging, 2020, 20(1): 98. doi: 10.1186/s12880-020-00497-w [15] Machireddy A, Thibault G, Tudorica A, et al. Early prediction of breast cancer therapy response using multiresolution fractal analysis of DCE-MRI parametric maps[J]. Tomography, 2019, 5(1): 90-8. doi: 10.18383/j.tom.2018.00046 [16] Fardanesh R, Marino MA, Avendano D, et al. Proton MR spectroscopy in the breast: technical innovations and clinical applications[J]. J Magn Reson Imaging, 2019, 50(4): 1033-46. doi: 10.1002/jmri.26700 [17] Baltzer PAT, Dietzel M. Breast lesions: diagnosis by using proton MR spectroscopy at 1.5 and 3.0 T: systematic review and meta-analysis[J]. Radiology, 2013, 267(3): 735-46. doi: 10.1148/radiol.13121856 [18] Bolan PJ, Kim E, Herman BA, et al. MR spectroscopy of breast cancer for assessing early treatment response: results from the ACRIN 6657 MRS trial[J]. J Magn Reson Imaging, 2017, 46(1): 290-302. doi: 10.1002/jmri.25560 [19] Choi JS, Yoon D, Koo JS, et al. Magnetic resonance metabolic profiling of estrogen receptor-positive breast cancer: correlation with currently used molecular markers[J]. Oncotarget, 2017, 8(38): 63405-16. doi: 10.18632/oncotarget.18822 [20] Gruber S, Debski BK, Pinker K, et al. Three-dimensional proton MR spectroscopic imaging at 3 T for the differentiation of benign and malignant breast lesions[J]. Radiology, 2011, 261(3): 752-61. doi: 10.1148/radiol.11102096 [21] Sippo DA, Burk KS, Mercaldo SF, et al. Performance of screening breast MRI across women with different elevated breast cancer risk indications[J]. Radiology, 2019, 292(1): 51-9. doi: 10.1148/radiol.2019181136 [22] Partridge SC, Amornsiripanitch N. DWI in the assessment of breast lesions[J]. Top Magn Reson Imaging, 2017, 26(5): 201-9. doi: 10.1097/RMR.0000000000000137 [23] Jena A, Taneja S, Singh A, et al. Role of pharmacokinetic parameters derived with high temporal resolution DCE MRI using simultaneous PET/MRI system in breast cancer: a feasibility study [J]. Eur J Radiol, 2017, 86: 261-6. doi: 10.1016/j.ejrad.2016.11.029 [24] You C, Li JW, Zhi WX, et al. The volumetric-tumour histogrambased analysis of intravoxel incoherent motion and non-Gaussian diffusion MRI: association with prognostic factors in HER2- positive breast cancer[J]. J Transl Med, 2019, 17(1): 182. doi: 10.1186/s12967-019-1911-6 [25] Suo ST, Cheng F, Cao MQ, et al. Multiparametric diffusionweighted imaging in breast lesions: association with pathologic diagnosis and prognostic factors[J]. J Magn Reson Imaging, 2017, 46(3): 740-50. doi: 10.1002/jmri.25612 [26] Kawashima H, Miyati T, Ohno N, et al. Differentiation between luminal-A and luminal-B breast cancer using intravoxel incoherent motion and dynamic contrast-enhanced magnetic resonance imaging [J]. Acad Radiol, 2017, 24(12): 1575-81. doi: 10.1016/j.acra.2017.06.016 [27] Ma DJ, Lu F, Zou XX, et al. Intravoxel incoherent motion diffusionweighted imaging as an adjunct to dynamic contrast-enhanced MRI to improve accuracy of the differential diagnosis of benign and malignant breast lesions[J]. Magn Reson Imaging, 2017, 36: 175-9. doi: 10.1016/j.mri.2016.10.005 [28] Park VY, Kim SG, Kim EK, et al. Diffusional kurtosis imaging for differentiation of additional suspicious lesions on preoperative breast MRI of patients with known breast cancer[J]. Magn Reson Imaging, 2019, 62: 199-208. doi: 10.1016/j.mri.2019.07.011 [29] Ye DM, Wang HT, Yu T. The application of radiomics in breast MRI: a review[J]. Technol Cancer Res Treat, 2020, 19: 1533033820916191. [30] Luo WQ, Huang QX, Huang XW, et al. Predicting breast cancer in breast imaging reporting and data system (BI-RADS) ultrasound category 4 or 5 lesions: a nomogram combining radiomics and BIRADS[J]. Sci Rep, 2019, 9: 11921. doi: 10.1038/s41598-019-48488-4 [31] Jiang XH, Xie F, Liu LZ, et al. Discrimination of malignant and benign breast masses using automatic segmentation and features extracted from dynamic contrast-enhanced and diffusion-weighted MRI[J]. Oncol Lett, 2018, 16(2): 1521-8. [32] Parekh VS, Jacobs MA. Integrated radiomic framework for breast cancer and tumor biology using advanced machine learning andmultiparametric MRI[J]. Npj Breast Cancer, 2017, 3: 43. doi: 10.1038/s41523-017-0045-3 [33] Fan M, Li H, Wang SJ, et al. Radiomic analysis reveals DCE-MRI features for prediction of molecular subtypes of breast cancer[J]. PLoS One, 2017, 12(2): e0171683. doi: 10.1371/journal.pone.0171683 [34] Xie TW, Zhao QF, Fu CX, et al. Differentiation of triple-negative breast cancer from other subtypes through whole-tumor histogram analysis on multiparametric MR imaging[J]. Eur Radiol, 2019, 29 (5): 2535-44. doi: 10.1007/s00330-018-5804-5 [35] Park JE, Kim D, Kim HS, et al. Quality of science and reporting of radiomics in oncologic studies: room for improvement according to radiomics quality score and TRIPOD statement[J]. Eur Radiol, 2020, 30(1): 523-36. doi: 10.1007/s00330-019-06360-z [36] Crivelli P, Ledda RE, Parascandolo N, et al. A new challenge for radiologists: radiomics in breast cancer[J]. Biomed Res Int, 2018, 2018: 6120703. [37] Leng XL, Huang GF, Zhang LH, et al. Changes in tumor stem cell markers and epithelial-mesenchymal transition markers in nonluminal breast cancer after neoadjuvant chemotherapy and their correlation with contrast-enhanced ultrasound[J]. Biomed Res Int, 2020, 2020: 3869538. [38] Fang C, Yang T. Value of tissue elastography in the prediction of efficacy of neoadjuvant chemotherapy in breast cancer[J]. J BUON, 2019, 24(2): 555-9. [39] Zhu Q, Poplack S. A review of optical breast imaging: multimodality systems for breast cancer diagnosis[J]. Eur J Radiol, 2020, 129: 109067. doi: 10.1016/j.ejrad.2020.109067 [40] Zhi WX, Liu GY, Chang C, et al. Predicting treatment response of breast cancer to neoadjuvant chemotherapy using ultrasoundguided diffuse optical tomography[J]. Transl Oncol, 2018, 11(1): 56-64. doi: 10.1016/j.tranon.2017.10.011 [41] Wei J, Wang C, Xie XX, et al. Meta-analysis of quantitative dynamic contrast-enhanced MRI for the assessment of neoadjuvant chemotherapy in breast cancer[J]. Am Surg, 2019, 85(6): 645-53. doi: 10.1177/000313481908500630 [42] Choi WJ, Kim HH, Cha JH, et al. Comparison of pathologic response evaluation systems after neoadjuvant chemotherapy in breast cancers: correlation with computer-aided diagnosis of MRI features[J]. AJR Am J Roentgenol, 2019, 213(4): 944-52. doi: 10.2214/AJR.18.21016 [43] Marinovich ML, Houssami N, MacAskill P, et al. Meta-analysis of magnetic resonance imaging in detecting residual breast cancer after neoadjuvant therapy[J]. J Natl Cancer Inst, 2013, 105(5): 321-33. doi: 10.1093/jnci/djs528 [44] Zhou J, Qiao PG, Zhang HT, et al. Predicting neoadjuvant chemotherapy in nonconcentric shrinkage pattern of breast cancer using 1H-magnetic resonance spectroscopic imaging[J]. J Comput Assist Tomogr, 2018, 42(1): 12-8. doi: 10.1097/RCT.0000000000000647 [45] Maria RM, Altei WF, Selistre-de-Araujo HS, et al. Impact of chemotherapy on metabolic reprogramming: characterization of the metabolic profile of breast cancer mda-mb-231 cells using 1h hr-mas nmr spectroscopy[J]. J Pharm Biomed Anal, 2017, 146: 324-8. doi: 10.1016/j.jpba.2017.08.038 [46] Pereira NP, Curi C, Osório CABT, et al. Diffusion-weighted magnetic resonance imaging of patients with breast cancer following neoadjuvant chemotherapy provides early prediction of pathological response–A prospective study[J]. Sci Rep, 2019, 9: 16372. doi: 10.1038/s41598-019-52785-3 [47] Suo ST, Yin Y, Geng XC, et al. Diffusion-weighted MRI for predicting pathologic response to neoadjuvant chemotherapy in breast cancer: evaluation with mono-, bi-, and stretched-exponential models[J]. J Transl Med, 2021, 19(1): 236. doi: 10.1186/s12967-021-02886-3 [48] Quiaoit K, DiCenzo D, Fatima K, et al. Quantitative ultrasound radiomics for therapy response monitoring in patients with locally advanced breast cancer: Multi-institutional study results[J]. PLoS One, 2020, 15(7): e0236182. doi: 10.1371/journal.pone.0236182 [49] Bian TT, Wu ZJ, Lin Q, et al. Radiomic signatures derived from multiparametric MRI for the pretreatment prediction of response to neoadjuvant chemotherapy in breast cancer[J]. Br J Radiol, 2020, 93 (1115): 20200287. doi: 10.1259/bjr.20200287 [50] Leithner D, Bernard-Davila B, Martinez DF, et al. Radiomic signatures derived from diffusion-weighted imaging for the assessment of breast cancer receptor status and molecular subtypes [J]. Mol Imaging Biol, 2020, 22(2): 453-61. doi: 10.1007/s11307-019-01383-w [51] Klimonda Z, Karwat P, Dobruch-Sobczak K, et al. Breast-lesions characterization using Quantitative Ultrasound features of peritumoral tissue[J]. Sci Rep, 2019, 9: 7963. doi: 10.1038/s41598-019-44376-z [52] Piotrzkowska-Wróblewska H, Dobruch-Sobczak K, Klimonda Z, et al. Monitoring breast cancer response to neoadjuvant chemotherapy with ultrasound signal statistics and integrated backscatter[J]. PLoS One, 2019, 14(3): e0213749. doi: 10.1371/journal.pone.0213749 [53] 杨少玲, 唐克强, 陶均佳, 等. 经皮下注射超声造影剂对乳腺癌前哨淋巴结的诊断效果[J]. 中国肿瘤临床与康复, 2017, 24(1): 10-3. https://www.cnki.com.cn/Article/CJFDTOTAL-ZGZK201701004.htm [54] Gampenrieder SP, Peer A, Weismann C, et al. Radiologic complete response (rCR) in contrast-enhanced magnetic resonance imaging (CE-MRI) after neoadjuvant chemotherapy for early breast cancer predicts recurrence-free survival but not pathologic complete response (pCR)[J]. Breast Cancer Res, 2019, 21(1): 19. doi: 10.1186/s13058-018-1091-y [55] Ming Y, Wu N, Qian TY, et al. Progress and future trends in PET/ CT and PET/MRI molecular imaging approaches for breast cancer [J]. Front Oncol, 2020, 10: 1301. doi: 10.3389/fonc.2020.01301 [56] Qiu XY, Jiang YL, Zhao QY, et al. Could ultrasound-based radiomics noninvasively predict axillary lymph node metastasis in breast cancer?[J]. J Ultrasound Med, 2020, 39(10): 1897-905 doi: 10.1002/jum.15294 -
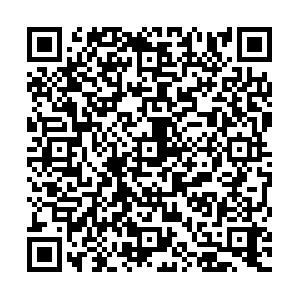
表(1)
计量
- 文章访问数: 300
- HTML全文浏览量: 171
- PDF下载量: 50
- 被引次数: 0